Research | PI: Yul HR Kang (강형률) | Teaching | Team | Contact
News
- Mar 2024: CoCo Lab’s very first Cosyne submission won Cosyne Presenters Travel Grant! Check out our abstract for the presentation led by Yeowon, “Uncertainty encoded in a recurrent neural network trained to predict visual input during navigation.”
Research
We compare how AI systems and the brain perform difficult cognitive tasks such as navigation, learning, and decision-making under uncertainty. We aim to use the insights gained to advance the mechanistic understanding and diagnosis of neurological and psychiatric diseases, such as Alzheimer’s disease.
We analyze neural and behavioral data from experimental collaborators and from the literature, and perform behavioral experiments ourselves. In doing so, we tackle the complexity of the tasks with tools from physics, machine learning, and robotics.
With excellent collaborators (below), we have shown that:
- Spatial navigation: grid fields (often called a “ruler in the brain”), which were thought to “deform” depending on the geometry of the environment, in fact represent uncertainty about the animal’s own location (Cosyne Presenters Travel Award; Bernstein Contributed Talk: video below). Here we developed:
- a normative theory that jointly predicts the deformation in the neural representation as well as bias and variability in homing behavior.
- an ideal observer model that takes as input the video from the 1st-person perspective and egocentric motion, and outputs the posterior belief over the agent’s allocentric location, which allows it to be applied to any setup without handcrafted features, as demonstrated by our re-analysis of historical results.

Preprint: https://doi.org/10.1101/2023.01.30.526278
- Episodic memory: memory of a unique episode is retained with a graded sense of uncertainty, which has not been considered quantitatively in the domain of episodic memory. We showed that not only is this uncertainty used in causal inference, as reflected in explicit choices, but also betrayed by gazes even after accounting for the explicit choices (CCN 2023).
- Dual-task: two decisions about one object cannot be made simultaneously; they are made one by one, and evidence for each accumulates in an interleaved fashion. We showed this by developing novel behavioral tasks & efficient drift-diffusion models, which fit the joint distribution of choices & reaction times (eLife 2021; Excellent Poster Award: Korean Association for Computational Neuroscience; Cosyne Contributed Talk).
- Conscious awareness: people become aware that they reached a decision when evidence for the decision is accumulated up to a threshold, which we showed by developing a novel behavioral task & analysis to predict & cross-validate the accuracy of the decision given the timing of the awareness using drift-diffusion models (Current Biology 2017; News piece in the Independent).

PI: Yul HR Kang (강형률)
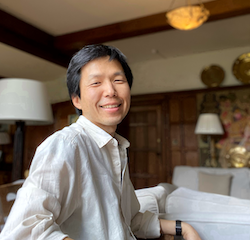
Education & Professional Appointments
- Assistant Professor (2023-), Department of Bio and Brain Engineering, Korea Advanced Institute of Science and Technology (KAIST)
- Lecturer (2022-2023), Department of Biological and Experimental Psychology, Queen Mary University of London
- Junior Research Fellow (2019-2022), Wolfson College, University of Cambridge
- Postdoctoral Research Associate (2018-2022), Computational and Biological Learning Lab, Department of Engineering, University of Cambridge (Máté Lengyel group)
- PhD in Neuroscience (2018), Columbia University (Michael Shadlen lab; Supported by the Vision Training Grant from the National Eye Institute)
- MD, Seoul National University, South Korea
- Summer/Winter Schools for International Olympiad in Informatics, South Korea
Collaborators
- Máté Lengyel (University of Cambridge)
- Daniel Wolpert (Columbia University)
- Michael Shadlen (Columbia University)
- Gergely Csibra (Central European University & Birkbeck University of London)
- Guifen Chen (QMUL)
- Hugo Spiers (UCL)
Teaching
- BiS427 Computational Neuroscience (Spring 2024, syllabus)
- AI Models of Brain Dys/function (Fall 2024, planned)
2023 (c) CoCo Lab